Study: Hybrid model to make weather forecasts cheaper and more efficient
For medium-term weather forecasts, AI is unreliable and conventional methods are costly. A hybrid approach should combine their advantages.
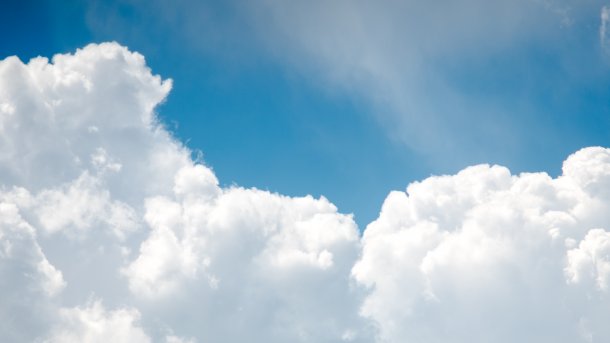
A hybrid model is intended to combine and perfect the advantages of AI calculations and classic weather forecast models.
(Image: Shutterstock/Milos Milosevic)
A combination of classic weather forecasting models (GCM - general circulation models) and artificial intelligence (AI) is to unite the best features of both approaches and lead to more precise results. This was reported by a research team from Google Research in an article published in the journal Nature. Previously, AI had already delivered good or even better results than the physical models for short-term predictions. However, AI was unable to achieve any improvement in ensemble forecasts with several possible scenarios and longer-term weather and climate simulations. The NeuralGCM model now aims to combine the advantages of the physical approach with those of artificial intelligence. Earlier AI approaches had already promised to be significantly more resource-efficient - for example in terms of energy requirements.
The model is competitive with AI models for forecasts of one to ten days and sometimes surpasses them, the research team writes, and it can also compete with the ensemble forecast of the European Center for Medium-Range Forecasts (ECMWF) for one to fifteen-day forecasts. The model can also reproduce climate metrics based on sea surface temperature for several decades. Predictions with a resolution of 140 kilometers could reveal phenomena such as a realistic frequency and path of tropical cyclones, the researchers said in the paper.
AI models only reliable for short-term forecasts
Traditional calculation methods work with values such as air pressure, temperature or humidity in the Earth's atmosphere and physical equations. In order to reduce the computational effort, the complex nature must be simplified. This applies, for example, to cloud formation, which also plays a decisive role in forecasting. The numerical approach has improved over the past 70 years, but has also required better computers for the calculations.
AI models such as GraphCast- developed by Google's AI company Deepmind - or Huawei's Pangu learn from historical weather conditions through reanalysis and calculate how the weather could develop. The models had difficulties with the ensemble forecasts: due to the atmosphere's characteristic of being a chaotic system, even small uncertainties can lead to major misinterpretations and errors in the forecast. The "ensemble" of predictions, which covers different parameters, is intended to cover this variance in the result. The further into the future the forecast should look, the greater the possible differences between the possible weather scenarios.
Further research needed
Compared to traditional forecasting models, Neural GCM is more efficient and less complex, writes the research team. At the same time, the researchers assume that alternative training strategies will be needed to understand important processes for the climate with subliminal effects on the weather. The team also sees the potential for hybrid models based on AI and physical models to find their way into other areas, such as materials research.
(are)